See FISITA Library items from Danilo Mendes Pedroso
EB2021-STP-015
Paper + Video + Slides
Abstract
Ing. Fabio Squadrani, Applus IDIADA, SPAIN
Mr. Danilo Mendes Pedroso, Applus IDIADA, SPAIN
Mr. Kenneth Mendoza, Applus IDIADA, SPAIN
Dr. Eng. Juan J. Garcia Bonito, Applus IDIADA, SPAIN
Mr. Juan Pablo Barles, Applus IDIADA, SPAIN
Mr. Antonio Rubio, Applus IDIADA, SPAIN
Mr. Antonio Jesus Contreras, Applus IDIADA, SPAIN
Mr. Jose Francisco Martinez, Applus IDIADA, SPAIN
Research and/or Engineering Questions/Objective:
The availability of big sets of data coming from brake durability tests paves the way for making predictions and decisions related to the noise coming from brakes. In this paper, the workflow for detecting brake squeal and all its main characteristics is presented.
Methodology:
Initially, a uniform set of data is generated, having a repetitive structure and format. This set of data will be used to train the machine learning algorithm. From the raw data coming from the vehicle data acquisition system, a spectrogram is mathematically generated, to graphically associate sound pressure level and noise frequency within the time domain. These spectrograms will be used to train the machine learning algorithm, which will be recognizing brake noise using the spectrogram images. The final objective is to detect squeal and to identify the frequency, sound pressure level, and subjective rating as well.
Results:
Once the algorithm is trained with thousands of brake noise events coming from real-life brake durability, brake noise is detected with a very high level of confidence. Currently, brake squeal is the noise being identified during this first phase of the project and is identified with a proper level of confidence, including frequency and SPL.
In the second phase of the project, the algorithm is also being evolved to associate a rating to the squeal noise event detected. The algorithm is capable to predict the subjective rating provided by a professional driver during standard driving or during specific noise research maneuvers.
Limitations of this study:
The real-time detection is currently under investigation and could affect the resolution of the spectrogram to be used to train the algorithm and to detect the brake noise. However, the current level of the study does not currently show any predictable problem that could arise when the machine learning algorithm is embedded within a real-time system.
Other brake noises should also be identified, even if less amount of data is available when compared with brake squeal.
Conclusion:
The study shows an alternative method for automatic noise detection and shows the possibility of automatically rating the brake noise. Real-time detection is also investigated and the results of its initial integration within embedded systems is shown.
EuroBrake 2021
NVHV
Downloads
Error message goes here.
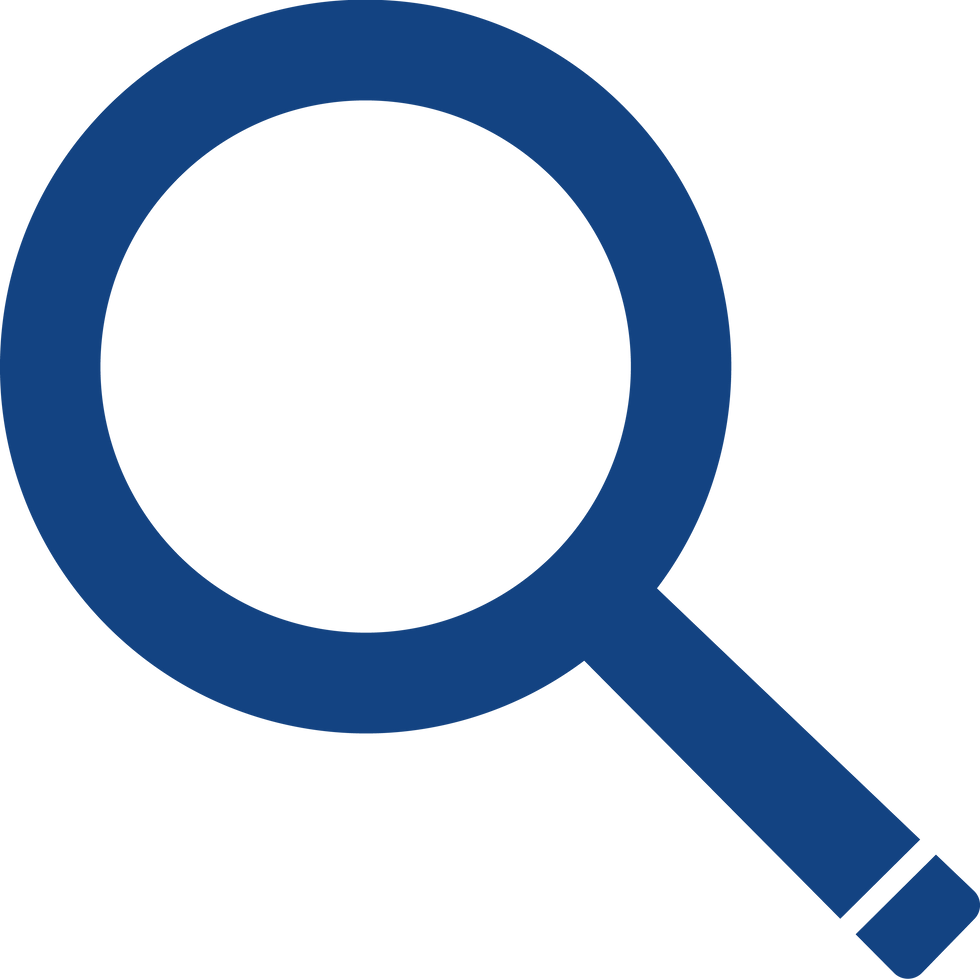
Person profile goes here.
Spain
Mr. Danilo Mendes Pedroso
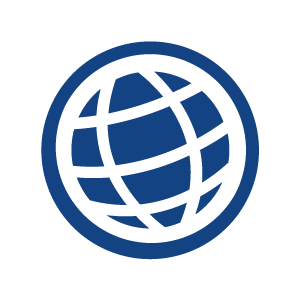
FISITA Committees & Groups
